Vidushi Sharma
IBM Almaden Research Center
Discovery and Optimization of Battery Materials in the Era of Foundation Models
With the rise of energy storage demand, the quest for efficient and sustainable battery materials has also intensified. Though artificial intelligence (AI) approaches have significantly accelerated theorization of new battery materials and device-level optimization to achieve better performance, their successful practical application is still thwarted by challenging synthetic realization and limited availability of usable datasets to train AI models. In this talk, we dive into the transformative role of foundation models, such as chemical large language models, in accelerating the discovery and optimization of battery materials. By harnessing the contextual chemical knowledge from large unlabeled molecular data, these models facilitate the prediction of material properties, exploration of novel compositions, and optimization of performance parameters. We demonstrate the scope of foundation model in driving electrolyte discovery for novel lithium metal battery based on interhalogen (I-Cl) conversion mechanism. Foundation model fine-tuned for downstream task of predicting electrolyte properties such as salt solubilities and redox potential perform significantly better than conventional graph-based supervised learning models. We further elaborate upon the customization of molecular foundation models to represent complexities of mixed material systems such as liquid electrolyte formulations. The constituent choice and their relative compositions create a very high dimensional design space that make finding optimum electrolyte formulation a ‘needle-in-a-haystack’ problem. Foundation models simplify this multi-variate design optimization problem with their pre-learned chemical knowledge and inherent generalizability. The new electrolyte formulation is realized and experimentally validated for relatively high cathode loading based on design principles learned by the model. The new electrolyte consisting of total 8 constituents improve the practical capacity of the battery by 20%.
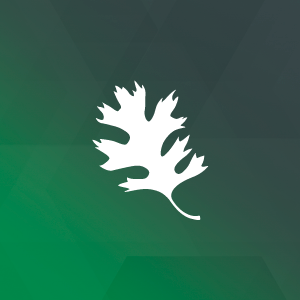